Machine Learning
Focusing on how computer programs can learn from and understand data, and then make useful predictions based on it, using insights from statistics and neuroscience.
Our research group focuses on how computer programs can learn from and understand data, and then make useful predictions based on it. These algorithms integrate insights from various fields, including statistics, artificial intelligence and neuroscience.
Machine-learning applications are increasingly part of every aspect of life, from speech recognition on cell phones to illness prediction in healthcare. One common problem is extremely polluted data, for which no single model can provide adequate explanations. At CWI we address this issue with statistical machine learning based on combining predictions from different models and experts in order to achieve reliable conclusions.
We also study how networks of neurons in the brain process information, and how modern deep-learning methods can benefit from neuroscience. We develop novel neural networks, like Deep Adaptive Spiking Neural Networks, and also theoretical models of neural learning and information processing in biology. Applications of our work range from low-energy consumption neural machine learning to neuroprosthetics, to increased insight into the question of how the brain works.
News
All newsERC Advanced Grant for Peter Grünwald for research on a revolutionary statistical theory
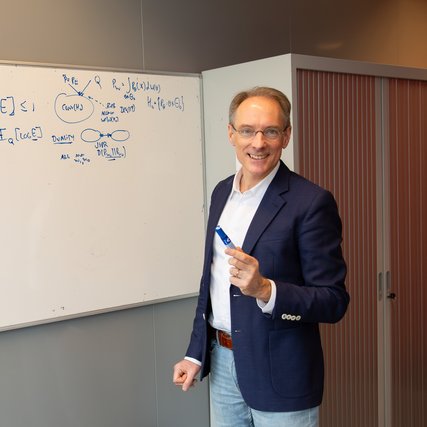
Making AI more energy efficient with neuromorphic computing
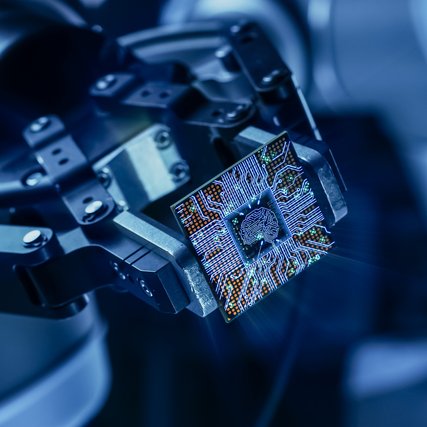
Revolutionizing statistics to tame the replication crisis
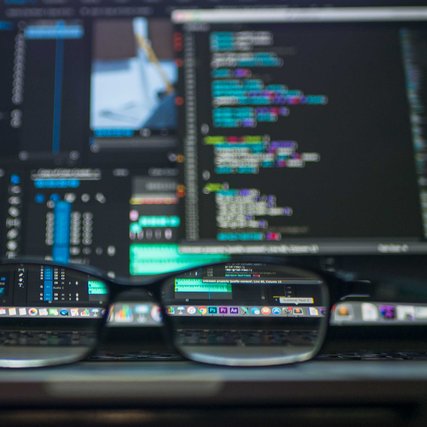
CWI involved in new consortium on energy-efficient AI
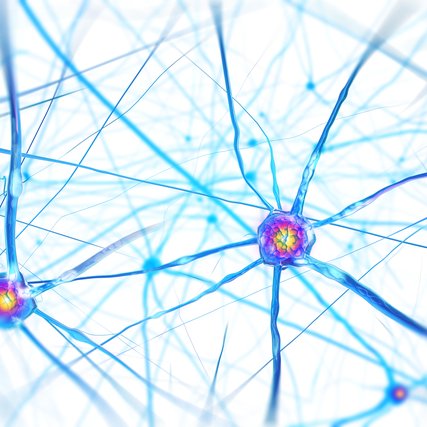
Publications
All publicationsCurrent projects with external funding
- Flexible Statistical Inference (FLEX)
- Efficient Models of Decision-Making for Asseing Cognitive Processing States (None)
- Increasing Scientific Efficiency with Sequential Methods (pre-proposal) (None)
- Perceptive acting under uncertainty: safety solutions for autonomous systems (None)