In the Scientific Computing (SC) group we develop efficient computational methods for systems with inherent uncertainties. The impact of these uncertainties (e.g. due to uncertain model parameters or to chaotic behavior) on model outputs and predictions is an important question in many applications.
We develop methods to model and compute with uncertainties in an efficient manner. Current research includes numerical algorithms for stochastic differential equations, Monte Carlo methods, uncertainty quantification, data assimilation, and rare event simulation. The computational methods developed in the SC group are aimed at applications in finance, energy systems and climate science.
Leader of the Scientific Computing group: Daan Crommelin
The SC group currently works on the following research topics:
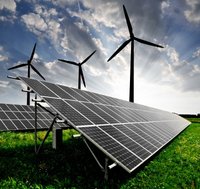
Uncertainty quantification and computational methods for energy systems.
This topic involves uncertainty quantification for assessing and reducing the impact of physical uncertainties in practical applications such as electrical grids, nuclear reactor safety, and atmospheric dispersion, together with industry partners. Furthermore, we develop numerical techniques for efficient quantification of failure risks in power grids, making use of rare event simulation methods. Senior SC reseachers: Benjamin Sanderse, Daan Crommelin.
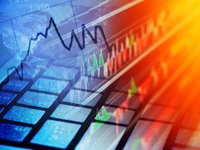
Computational finance.
Here, the focus is on economic decision-making and financial engineering. The latter topic is at the intersection of numerics and stochastics. We work on the highly efficient computation of so-called Backward Stochastic Differential Equations, and study their applicability in finance and insurance. Furthermore, we develop numerical algorithms for dynamic optimization of financial as well as other real-world portfolios, like portfolios of power plants, etc. This latter research is based on our newly developed Monte Carlo algorithms. Senior SC researcher: Kees Oosterlee.
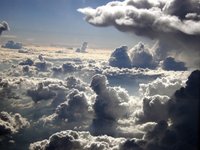
Data assimilation and stochastic modeling for climate, atmosphere and ocean.
Research on this topic has two themes. Data assimilation is an approach for incorporating data from observations into dynamical models for (e.g.) climate or atmosphere. We investigate the data assimilation methodology of particle filters and their use for reconstructions of the past climate. The second theme is the development of stochastic models for small-scale atmospheric and oceanic processes (e.g. cloud formation). Numerical models for global atmospheric and oceanic flows are too coarse to resolve all relevant small-scale phenomena. We develop stochastic methods to represent the impact of these so-called subgrid-scale processes in numerical models. Senior SC reseachers: Svetlana Dubinkina, Daan Crommelin.
(pictures: Shutterstock)